What Is AI And What It Means For Your Business?
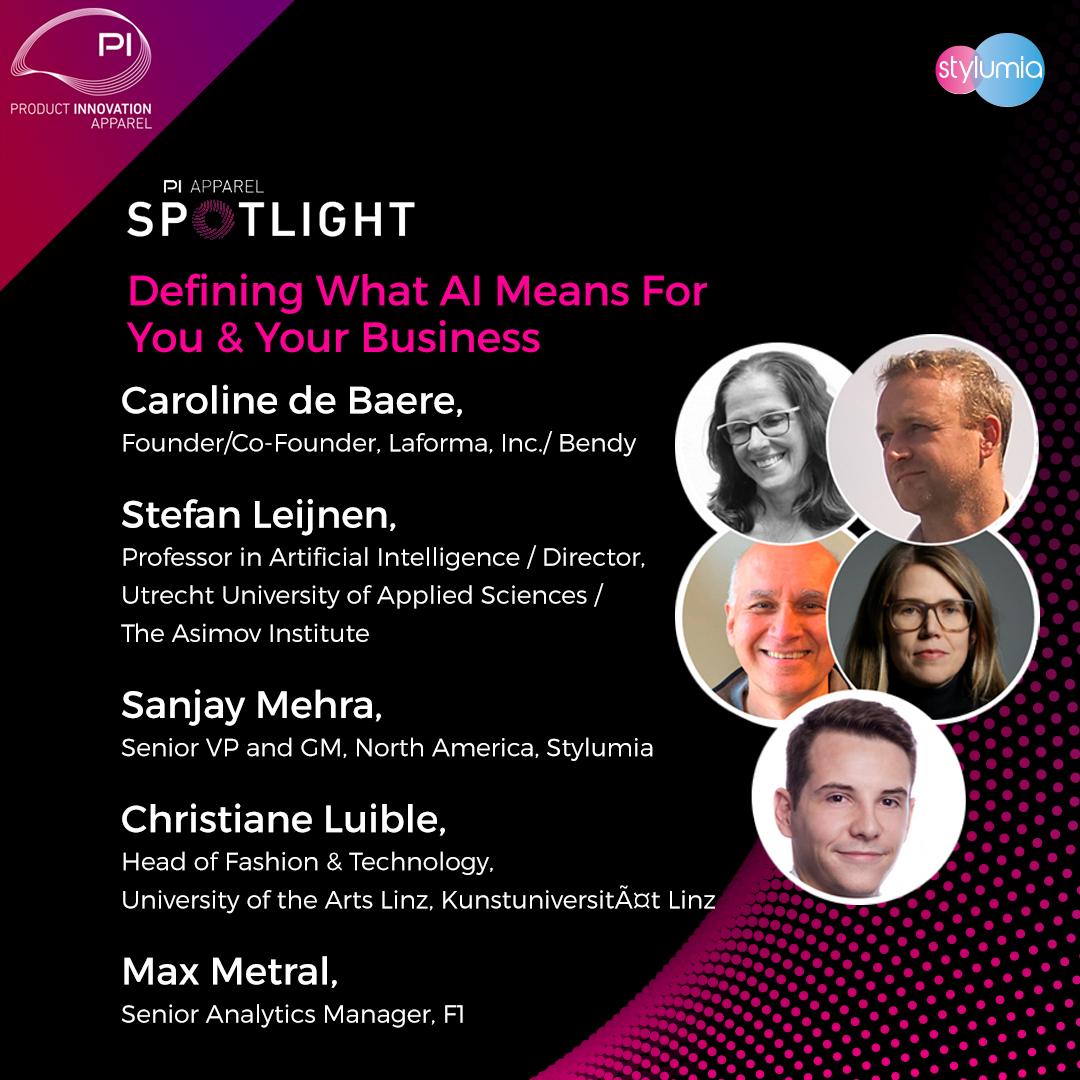
An expert panel from Formula 1, Academia and Stylumia discussed recently in a global webinar organised by PI Apparel. The topic was fundamental and profound “What is AI and what it means for your business?”
We present the full recording of this webinar and transcript below.
The full transcript of the video:
Stefan Leijnen:
Welcome, all to this panel discussion on defining what AI means for you in your business. I think this topic invites us for a lively discussion. So for those of you online, please join in as we discuss this theme and ask more questions. And we’ll try to dive in on those, to drive the discussion forwards. So I think a good way to start this discussion is by framing what we mean when we talk about AI. I took the liberty to look up the definition of AI by AU High-Level Expert Group. So let me start with this. So AI refers to systems, according to them, that display intelligence behavior by analyzing their environment and taking actions with some degree of autonomy, to achieve specific goals.
And what I’d like to do as a way of introduction of this panel is to ask the panellists not only to give a short introduction of themselves and their backgrounds but also to share their definition or their view on what AI is, with the audience. And maybe, we can start with you. Would you like to pick this up?
Camilla Olson:
Sure. My name is Camilla Olson. And I’m the co-founder and CEO of Savitude. And we bring speed and analytics to fashion design. For me, AI is a set of algorithms that with training is capable of learning.
Stefan Leijnen:
Thank you. Sanjay, would you like to go next?
Sanjay Mehra:
Yeah. Sanjay Mehra. I have about 25 years of experience in the apparel footwear space. And that’s been across technology, across analytics, of process, of strategy, supply chain and general management. My current role is the SVP and GM for Stylumia for North America. And we apply machine learning-based solutions to all aspects of decisions making across the value chain for the apparel business.
Stefan Leijnen:
Thank you. Max, would you like to introduce yourself?
Max Metral:
Yeah. And thank you very much for having me here, as I guess another industry expert. My name is Max Metral. I work as a senior analytics manager for Formula 1. So they’re outside of the apparel industry. And for me, so I work around … OR every business analytics subject. And that related to the core data, in case you ask. And so for me, AI is very interesting. Obviously, I’m not sure I can do a better job than the first definition you gave, Stefan. But for me, it’s just how you can use computers and machine learning, very much like Camilla explained. But to mimic human functions. So that’s why you can use it as face recognition, image recognition, language translations, all these things. I think for me the main benefit of AI is to speed things up and to have computers do things that humans could do, but is just too painful or too difficult to learn to do, a little bit more efficiently. And that would be my definition.
Stefan Leijnen:
Thank you, Max. And then finally we have Christiane. Could you also give an introduction of yourself and how you think about what AI is?
Christiane Luible:
Hi, my name is Christiane Luible. I’m the scientific person here. I’m not an AI expert. I’m a professor at the Fashion and Technology Department of the University of Art and Design in Linz. So I have a background in fashion design, but also in fashion research. So we focus a lot on research projects, fashion and technology. And we experiment with how we could use robots for design. So AI for me is a kind of extended machine learning. But just the focus of what can you really do with it? So how can we really learn and do better processed with these new possibilities?
Camilla Olson:
Sorry, if I could-
Stefan Leijnen:
Thank you.
Camilla Olson:
… ask. Stefan, if I could adjust my definition since others took liberties. I was brought up Catholic, so I’m very strict in how I follow instructions. And so I also agree with Sanjay. We use machine learning and a lot of visual recognition as well. Christiane, I can’t wait to talk with you a little bit about how we’re viewing it with fashion design.
Stefan Leijnen:
Yes, thank you. Unfortunately, Caroline couldn’t join us. She’s not feeling well today. So that makes me the final odd panellist. I’ll quickly introduce myself as well. So I’m a professor at the Utrecht University of Applied Science. I’m also director of The Asimov Institute where we research AI and creativity. So I have a background, a technical background in AI. I want to start with maybe a question that focuses on the value of AI to your business. I think you audience would be interested in that. So maybe if one of you wants to start and pick this up, in what ways can AI add value to your business?
Sanjay Mehra:
I’ll go.
Stefan Leijnen:
Sanjay, yes.
Sanjay Mehra:
Thanks, Stefan. So as was said by Max and others, there are several ways in which data can help augment decision making. And the key area that I always suggest for customers and partners is, where are those areas where you have a lot of data, and the data can provide you with rich insights? And how those insights can not only help augment decision making, and also optimize? And so some examples can be for instance, what is the right product? If anybody has gone onto a website and they’ve seen something that says, “Recommended for you,” there’s rightly a machine learning engine behind that. Analyzing root causes for why your business may be accelerating or not doing so.
Obviously optimizing for the highest revenue and margins. How do you manage pricing, particularly in a B2C type of business? Things like fraud detection. If you’re seeing a lot of traffic and there’s a higher incidence of fraud, of course, it’s a great AI and machine learning application. And so on. So you can look at this across the creative side of the business. You can look at the operating side of the business. You can even look at the organizational aspect of the business. Where will you have a good amount of data is a potential opportunity to get to a better place.
Stefan Leijnen:
Thank you. Maybe Camilla, you would like to from your perspective from Savitude, and where AI adds value to your business.
Camilla Olson:
Yeah. So I’d like to give a little … So I spent longer than I’d like to admit in the pharmaceutical industry as a serial entrepreneur. And I’ve found two predictive modelling companies. Back then we called it predictive modelling. One was simulating clinical trials. So I would call that pretty scientific there, analytics. And I had found myself somehow going back to fashion school, and I did an MFA in fashion design. Never wanted to do it. But hey, there I was. And running my own fashion label. And had a problem with fitting clothes on people. And realized that we could actually solve this fit problem using similar ways that we had solved clinical trial design.
So we started working on this. And we built a recommendation technology system using the knowledge base, correlating silhouette and design details with the curvature of the body. And with a lot of machine learning wrapped around it. And very a quite elegant visual recognition, robust visual recognition technology. But what we … So we made recommendations. So we’ve found an 11% … Quite a good recommendation system. But what we’ve found is that through this, is that there are pockets of people who we couldn’t recommend clothes for, because nobody had designed for them.
And so we realized that the problem is really back in the design studio, back in my neighbourhood. So then we had to take the same thing we built, and apply it and make an AI design product. So that to help fashion designers design for more body shapes. And because I was a designer, we approached it to assist, not to replace.
Stefan Leijnen:
Thank you for that perspective. I think it’s something that’s common in digitization where you’re brought in as the experts to help digitize existing business processes. And you end up changing the business processes themself and giving insights that were completely unexpected. I think this provides a very nice example of this. Perhaps Max, I can ask you to give your perspective, with respect to Formula 1.
Max Metral:
Absolutely. And I think they’re a lot of bridges that we can build between F1 and the apparel industry, and to be on exactly what Camilla and Sanjay said. I think we use AI on the business sides, I’m not talking about cars, in four different ways. I think of very similar to what you can do in the apparel and design industry. First is CRM. It sounds very obvious, but managing [inaudible 00:10:08] data or customers data. Which on our hand is very different. Not all our friends are customers. Nor that is our ambition either. That obviously there are things you can do, segmentation clustering, understanding better next best action models. And predictive, depending on if it’s a product you’re trying to sell. We have some products that are more like Spotify models. So pay by the month or annually. And so you have trained prediction, train management. And that’s one part.
Then the other products, which on our side, is we’re using data and AI and [inaudible 00:10:43]. More importantly, we scan and we try and understand the sentiment online of our products. Our product being F1 race. Does that mean doing the Grand Prix or doing the quali. And we really try and analyze what. And try to correlate this with what’s happening on the track.
The important things here that we’re trying to do, which I think can be very relatable to the apparel industry is trying to differentiate what people say they want, and what they really want, or what they like. What they say they like. We do some really cool stuff where we do some passive and active measurement. So for instance, passive would be, if you have a specific application on your phone and you ask someone to click when there’s something they like when the race is on. Versus passive, which is you monitor Twitter, or you monitor some online forums that are readily available, and like and don’t like. And sometimes you see some very big differences in what they say they like. Where actually when it happens on T.V. it doesn’t impact much of the engagement, or it doesn’t impact all the KPIs we’re trying to measure. So that’s I think what’s interesting on the product side.
We do have digital. And I don’t include this in CRM, because digital is slightly a different type of data, cookie base, still anonymous. There are things that can be based on us to improve our traffic. And as Sanjay said, there are a lot of things you can do in there. People like you, like this content or this video, or obviously this product in the apparel. And lastly, we do have things around pricing. And we have different products that we sell, where that’s obviously still relatable to the apparel industry with your pricing and. We do pricing on our OTT offer, which is F1 TV. So when we sell that to consumers. We do pricing on sponsorship. Different type of products. May not all be relatable to the apparel. But obviously, pricing analytics is a big thing. What’s a good price? And how we can also try to help. So help decision making within the business. So that’s the different ways we use AI and business analytics in the company.
Stefan Leijnen:
Thank you. Christiane, perhaps you have a wide overview considering your academic perspective. Could you also offer a perspective on this question what AI can add to business value in general?
Christiane Luible:
So we are focusing at the moment not so much on the data side, or in this input and the data segment. For us, the focus is pretty much on how could we inform the design? So we have an ongoing research project at the moment called Fashion and Robotics, where we try to capture what we’re actually doing as a designer. So what do I do as a designer? I motion track what I’m doing. I transfer this to a robot, and I see how much interpretation could I get here to obtain a new design. Because I think if you want to create really something new if you also integrate something called the zeitgeist, and the social side of fashion. So if we’re really looking in the future, I think it’s very complicated at the moment. So at the moment, we are really looking, “Okay, what we are actually doing as a designer.”
So what we are doing as a handcraft, as draping. And so how would a robot interpret this? And so in a kind of generative design. So what would an intelligent machine propose as a design? So it’s not data-based, it’s not customer based. It’s more on an expertise-based. On, “What is my profession? What am I doing?” So that’s not easy. It’s the first steps.
Stefan Leijnen:
Yeah. Do you find that when you’re looking into a sort of creating these robots or these software algorithms that take over a part of this creative process, do you find that most designers have similar creative processes? Or is it different every time? Because I can imagine if you’re trying to automate part of this process, that some type of similarity in the process is very helpful.
This is a very good question. And I think I can better answer it maybe in two years. Because we are just at the start of the project. And at the moment, we are just happy that we can somehow track and transfer the movement to the robot. We are very happy that, not in real-time, but the robot is doing what I am doing. And then the next step would be a kind of machine learning. So, “Okay, what do you do, and how would the robot do it?” So I think this is a field where I think for thinking in businesses, I think it’s quite a step to go, I would say. But this is our … It’s our outlook for the future.
Stefan Leijnen:
Great. Thank you.
Camilla Olson:
I can actually answer that question from a different point of view. Because we’re solving the same problem from a different perspective. The way I learnt is design at fashion school is to take inspiration, trend, and design for there. And incorporate that into a new design. And making sure that the new designs are within brand DNA. And that’s how we’re designing. But we draw new designs in our system. So we actually are able to draw it so that it … Since we capture the archive of the design house of the designer, what we draw looks like what the designer has done previously.
But it is challenging to try and talk to designers about this. Because our goals … Because if you read the headlines, you know lots of creatives are quitting, because … I’ll tell you, that was the hardest job I ever had was being a fashion designer. It’s exhausting. But we’re gluttons for punishment. We built this to help them, but they don’t really want help. And it’s hard to convince them that this is not cheating, and this sort of thing. So we’re early days. We’re just beginning to launch the product. But it’s challenging to bring technology into creatives.
Stefan Leijnen:
Yeah.
Sanjay Mehra:
Yeah. I just want to add to what Camilla just said. And at Stylumia, we’re also taking insights from website crawling. So for instance, what is the style trend, what’s the color trend? What’s the trend of the collar? Is it of a particular shape, et cetera? And we’ve turned that what you’re describing, into also … And this is again based, a generative adversarial network-based engine that does come back with designs. What we’ve found is that just presenting that as a thing to play around with, it was actually much more helpful. Because there’s no way that we’re looking at replacing the designer. Right?
But what we are showing and demonstrating is that “Hey, this could actually help with the designer’s inspiration,” as you’ve described. And so that is an area we’re seeing a little bit, I would say more openness. And also a wow factor. It’s like, “Oh, I didn’t know that you can actually do this with machine learning.” And the point that you just brought up, Camilla, around adoption. I think that’s the toughest challenge in this journey. And even when we found teams that are well versed with the change, and believe they’re technically savvy. The idea of presenting a black box that is called a machine learning engine. The black box is putting out some output and some recommendations. And that is a tough one. And we can maybe talk about it more a little later. But the human side of AI adoption is, I think the most critical aspect of the journey.
Camilla Olson:
And so here’s the thing. So I’m in Silicon Valley where Digidesign was started. And that’s where they brought in technology to music creation. And I shared my husband was in the startup when it was starting in the early days. So I talked to Peter Gotcher who is the CEO. And they also took really 10 years to get that technology adopted. And it was not AI. They went into the garage, and they sampled all the different instruments and put it together to help musicians. But it really was a helping tool, that it was technology. That was a black box in those days. So we’re just at the new frontier of a new kind of black box, I guess. But I’m hoping that it will be less than 10 years for adoption on this one.
Stefan Leijnen:
Well, talking about adoption. I can imagine if I were a fashion designer who is worried that my job would disappear, or would be alternated. But I would have knowledge of AI and data. I would probably bring forth the following argument. What we see in AI is that it’s basically statistics, particularly the type of AI that has become popular over the last decade, looks back into historical data. And tries to infer or extrapolate from that data into the future. And so this will be my argument as a fashion designer, “How could I ever be replaced? Because I’m capable of looking forward instead of looking just backwards.” What would you say to this fashion designer?
Max Metral:
I’m happy to take that one. Not being a fashion designer, maybe I get the benefit of the doubt. I’d say that for me, AI or computer machine learning what I’m going to use. It works the exact same way humans work. We base ourselves data points with the information we have. Whether we accept it or not, whether we’re fully aware of it or not. And there you can take into account some biases or so that sometimes we’re not aware of. But we do sometimes also … We do always make decisions. And I think creativity is just a different way to rearrange things we’ve seen somewhere else in another way. But I agree with you. The point I guess isn’t to replace people. It is to help them in the best way possible. So I think that’s where I would say, it’s like, don’t be afraid.
Christiane Luible:
I can add something also to the discussion before. Because adoption in design, I think there’s also a new generation of students coming now. And if you come in the education over the project, and then if they really feel that there is a need, or if it makes sense for their artistic project, they’re quite good in learning technology. This is our experience here in Linz. And I also agree with what you say, this historical data. I think fashion design if you really think of new and the social aspect of design, I think it’s a very complex thing.
We are a very complex society, what we interpret in fashion design. So obviously we can not base a zeitgeist today on data. I think there is more to really generate a new design. I think at the moment, we can create something. But we are not really creative in the sense with the machines, or with machine learning. But it doesn’t mean that it’s possible. Because in fashion design, we are much more confronted with data what we have to consider. I just bring in the of sustainability. I think we face huge challenges.
So why not informing this AI to make better decisions? To have a better design based on better decisions. And I think here today it could already do a lot in informing better. And that step by step, we will see. I think today we just do not know when it comes that we say, “Oh, yeah. This is now really a good design made by AI. This is really future.”
Camilla Olson:
Christiane, I think you’re right on the money there. And I think that we have been talking about what’s wrong with fashion. And fashion is going through so much turmoil. And I think COVID just exacerbated the issues with fashion. And so I think AI and for example, our technology, and Sanjay’s technology, and many other technologies, are facilitating a transition into future fashion. And one of the things that we do, and I’m sure many other companies, can help reduce everything that’s going into the landfill, and other pollution issues. And inequity. So many social problems that we can also address new ways of looking at fashion. And so I think we were talking about new technologies, we should be looking at maybe bringing on new business models to solve more than one problem. So that’s how we’re looking at it, and we’re embracing. And a lot of people are talking to us about new business models. And going forward, we’re solving many of these problems.
Stefan Leijnen:
But that’s actually-
Sanjay, yeah, go ahead. Yeah.
Sanjay Mehra:
Sorry. Thanks, Stefan. If I can just add some context or some data to this. So recent studies have shown that there are over 100 billion pieces of apparel produced every year. And just about one third really hit the mark in terms of the right style, the right price, the right fabric, et cetera. The right color, right? And the rest of it is this long tail of discounting and promotion. And a huge number of these garments actually go into landfills and to donation centers, and other places that were not intended right from the get-go. And while I think there is a general awareness about how AI and machine learning can help this problem, I think there are many aspects all along the value chain where we can create the solutions that help companies and organizations get more precise with the decisions they’re making. And therefore reduce the environmental impact. So totally agree with what you both said, Camilla and Christiane. It’s a superb opportunity in front of us.
Stefan Leijnen:
Yep. Thank you for that perspective. Talking about business models, I’d like to make a leap to the next question. So looking at the AI ecosystem and how it’s developed in general, also outside of apparel. We’ve seen many large companies and industries jumping on the bandwagon very early. Particularly big tech. So we know Google, Apple, Facebook invested heavily in talent, and also startup acquisition. For example, Deep Mind has been bought by Google for over $500 million in 2014, six years ago. Now recently, we see that AI technology is becoming more and more commodified. Accessible to a wider audience of companies. What I’d like to ask you is, to what extent do you believe that AI is viable for any company size?
Max Metral:
I’m happy to start with that one. I think there is a very interesting saying by Dan Ariely. He’s a professor of Behavioral Economics at Duke, I believe. He said a couple of years ago, it’s not new. Something like, “Big data is like teenage sex. Everyone talks about it, nobody really knows how to do it. Everyone thinks everyone else is doing it. So you have to claim that you’re doing it.” There is a bit of that.
Camilla Olson:
Wait a minute. Okay, let’s not take a poll. Sorry.
Max Metral:
Go on, Camille.
Camilla Olson:
No, I’m sorry. Oh, no. I’m sorry. I’m just being inappropriate.
Max Metral:
Sorry. No, for me what I wanted to say is, a lot of people claim they’re doing things they may not be doing. That’s my point. And when you look into the root of any company, it’s never as promising or as beautiful as they claim to be in the media. I think that’s I think the first thing is, you have to consider what you want to do yourself, rather than trying to do what people are claiming to be. I do personally believe it’s valuable for any company size. Despite how big F1 seems on the outside, we’re not that big. We’re quite a small company, in terms of especially the number of people. And we have to do things we can internally with what we have. And we don’t run on amazing budgets to do everything. And so we have to make decisions as to what can we do ourselves, what do we have to outsource? And we have to be smart about it.
And as Sanjay said, you have to analyze on the value chain what makes more sense for what we’re trying to do, work in AI, but be better than a human doing it? Or where it makes no sense. And I think that’s … I believe you can use many tools. And nowadays they’re free to use or very cheap, from both data processing, data hosting. Just running models. You can use software that is totally free based on community. And there are many things you can do on the cheap, and be doing a really good job without having to go to the super expensive tools or models you can buy from whatever company. I think that’s my perspective. It is possible. It’s not easy. But I think it’s also about the people. And I think it’s also about how you want to bring in. And as all the panel has said before, the human challenge is the biggest one, more than the technological challenge. And about forming people internally, helping for a transition if it changes the people’s job for people day-to-day. And this is for me what’s important more than how expensive it is to do it.
Stefan Leijnen:
Thank you for that perspective. Any other of the panellists would like to comment on this question about AI being valuable to any company size?
Sanjay Mehra:
Sure.
Camilla Olson:
Yeah. I think that if you’re talking about this very small company developing their own. Or even a lot of people doing it in-house. I think that that’s getting to the point where that’s maybe not a good idea. There are so many companies building really, really strong solutions for everyone. There’s got to be standards. Because if you want to grow, there are going to be other things built for the standard. You’ve got to start buying from those company who are supplying the solutions. So yeah, going a long way to say, I think everybody can use AI. But I think buying it from people who know what they’re doing when they’re building it is probably the better way to go.
Sanjay Mehra:
… if you think about the fundamentals of what it takes to take advantage of AI, it’s the ability to model a problem. And it’s the availability of good data. And I can’t stress the data aspect enough, right? So a small company, a startup, two people, one person, absolutely you can take advantage of these capabilities. Because there’s somebody else likely, to Camilla’s point, that’s providing that solution. So I think it’s about the mindset.
The other is, the number of times that I’ve seen challenges in this industry, I’m going to give you very specific examples. Does the organization have a consistent definition of what a style is? Right? There’s a lot of data out there, but the ability to get clean data, the ability to have data that doesn’t have any duplication, data that can be integrated across different dimensions. That is kind of the gas for the engine. So if you think about an F1 analogy, you can have the very best engine. But if the quality of the gas is not at par, then you’re not going to get good results.
And so that to me is part of the consideration. But if you’re looking at external data as a startup or a small company, then you have to ask the provider what have they done to make sure that the data quality is good. And if you’re looking to embark upon this journey internally, then I think having the right standards, the right guidelines, and the right strategy around how to use data as an asset, will go a long way.
Stefan Leijnen:
Yeah. Sanjay, you have a background in VC. So this lock-in situation that can occur, right? Where if you are an early-stage startup, you need data. Sometimes you have a launching customer that will provide you with this data, but it might not be so happy if you find another customer. What advice would you offer? And I know that this is a difficult question. But what advice would you offer to early-stage startups, or maybe people thinking about being an innovator in this field, on how to deal with this data scarcity problem?
Sanjay Mehra:
So there’s a couple of things. I think one is, a lot of companies as they’re thinking about what would be an information-centric operating model, right? I’ll start there. And I’ve seen this with a lot of customers. What I mean by an information-centric model is thinking about data first before you think about the process. A lot of companies have been designing by thinking process first, and then how does data enable the process? So the first step I believe is to flip that.
And the second step then what happens is, is companies realize that there’s a certain area of their business that they believe they can actually create a differentiated advantage in. So there’s a competitive advantage for them. And so picking those areas I think is important. Because then you can start to invest to say, “I’m going to get my data ecosystem to be better than somebody else.” And then the third step I think typically is looking at what sources of data you have. Because chances are, there’s a lot of external data that can help you get going. Even though you may not have the scale and the amount of data internally. And so the type of decision making that you want to enable through AI and machine learning, and the bigger journey can be mapped in a way that you get some initial wins. And then you continue to evolve.
Camilla Olson:
Yeah. Can I … A point here. I also was a venture capitalist back in the 1980s in New York for four years. And I agree with some of you what said. But I have a slight diversion. I think it’s imperative that you have domain expertise when you gather your data. Otherwise, you have generic data, undifferentiated data. And that’s really what set us apart from the 200 other companies that are out there. So we sat down, we have fashion designers sit down with our engineers, and they’re still sitting down with our engineers. And so we built our own training sets.
And what that means is that because we had our own fashion label, we had our own photoshoots. And we know what the tricks are when you take photos. So our visual recognition datasets have… We make sure they have all those tells in there so that when we found things, we know how to identify them. And so we can go through social media and identify clothing in crowds, and when people are sitting on the side or clothing. Which other people can’t do. And that’s because we had the domain expertise in there. We didn’t know it then that that was going to be our advantage. But we know that today. And so I think that having the domain expertise from the get-go is really, really, really important. Because you don’t know what you don’t know. That’s going to be important later on.
Stefan Leijnen:
Thank you. So Christiane, perhaps you could offer your perspective on this viability of AI for company size. And maybe in answering the question, who do you think your research or maybe what companies your research will benefit. Will it be the larger industries, or maybe startups, or even students who are thinking about moving in this field?
Christiane Luible:
Obviously, we have no company experience. But in general, what we are doing in the university, we focus a lot on small sizes. We really want to make it possible for our students to create their own structures. And not necessarily joining a big company, but also to make it again, possible to start a business, a small business, startups. So every technological developed what we do, or invention what they are also developing together with us, we really focus on new technology, but affordable. So I also think what … Even if you work with the robot, it’s not necessarily highly expensive. There are also today affordable small robots. And I also believe a lot that as I mentioned before, we are not so much working with data. We have a different experience.
And this interface with the robot, I think this can especially augment the designer. It’s not replacing designers, it’s working together. It’s assisting. It’s, “How do I communicate? Maybe the robot is today doing tasks which are too heavy, or which are too complicated. Or which are too time-consuming.” So this working together, these collaborative processes, I think this is very much of interest for us and for finding new businesses, or finding new solutions with the students. And also with the data as we are at our university, we are also kind of critical in that sense. Because if you use too much data of the existing data, you base yourself also so much on existing reality. And I think today we also implement a lot this critical view on technology.
Like is the machine really doing it in a democratic way? Or is it doing it in an inclusive way? And these are also very important aspects what we are doing. And I think here also again, there’s a big potential for our students to create their businesses in the future. It’s more abstract. I mean, you are more in the business of today. But I think in the future, all these aspects what we try to educate will be important.
Stefan Leijnen:
Yes. I recognize what you’re saying also from my university. These things are very … I mean, we foresee these things becoming important in the next five to 10 years. We have a question from the audience I think which relates nicely to what we’re discussing right now. Which is particularly aimed at those of you with a background in other industries than apparel. So having experience in AI investment yourself, what is the first step for starting this journey? I think we already discussed the role of data or the importance of data in this first task. But perhaps you can take the wider look, and give advice to new people and new companies entering this field. What is the first step they should take?
Maybe Sanjay, yes?
Sanjay Mehra:
So first off, I think it’s recognizing that any new startup is going to have an aspect of data. That dimension of what data means to the business is a question I think that any business in today’s environment should be thinking about. I think the second is around how that data can provide meaningful use to the company. So we all live with data. But I think the ability to think about how that data translates into better decision making, or decision augmentation around masses of data that you couldn’t get after like Max was saying earlier.
The human, your capability just isn’t there. But you’re a startup, and you want to see that ability. Well, that’s another example. I think the third and very important one and Camilla can talk about this is, who in your company, if you are the founder and the sole individual, you’re also wearing a CDIO hat, or a chief data information officer hat, right? So at the very early stage, who is the talent that you are bringing in, that can think about this new paradigm? And that can bring that thinking into the core operation of the business. I think those are some examples. There are so many different ways to slice and dice this. So I’d be the first to say that this isn’t the only one. But that’s just a thought.
Stefan Leijnen:
Camilla, yes.
Camilla Olson:
Yeah. I think the first thing you need to do is talk to your customers, and make sure there’s a need that you’re going to be able to sell your product. And you should develop relationships, from a customer advisory board to keep … Not a formal thing. But keep … Start a community. Keep that engagement going, or you’re going to be building something, and that … A bridge with no landing. People tend to fall in love with what they’re doing. And like we … Yeah, yeah. Too many times that happens.
Stefan Leijnen:
Which capabilities do you think are essential to have in place, in order to ensure AI’s success? So we have the data, we have the customer, maybe access to capital as well. But which capabilities or type of talents should.
Camilla Olson:
Well, you kind of just threw away that access to capital. That’s not so easy.
Stefan Leijnen:
Okay. Go ahead, yes.
Camilla Olson:
It’s not … Yeah.
Sanjay Mehra:
I think the biggest quality is curiosity. If you’re willing to ask yourself the question, “How else could I solve this problem? And how could I apply a different way of thinking?” Then that’s the first step. Because it helps you discover what capabilities are there that one may not even come across.
Max Metral:
I think-
Camilla Olson:
And persistence, yeah.
Stefan Leijnen:
Max, I think … Yes?
Max Metral:
Yeah. I totally agree with Sanjay and Camilla. Curiosity and persistence. I think to add to this, I feel one of the first thing which is very important to get it right is getting the right person to lead this AI transformation, or data transformation, however, you want to call it. Some people call them data translators. I’m not a big fan of the name. But I think that the position is interesting itself, is they define this as people that are business savvy. So they understand the business they’re involved in. So for me, it would be the sports industry or the apparel industry. They know all the different type of jobs and what people are doing in the company.
And they can clearly identify where data can help. And that’s not that easy to do, because you have translated a business problem into data problems. And then once they have the data answer, to transfer this back into actionable accommodations. Which is a very difficult job. I think getting this quality, and this curiosity is very important. More so than getting a data engineer, who is still very valuable. But I think getting a person who can talk business, and understand the value of the business and everyone in the company, and translate this into data problem. And leading a project, I think this is very key.
Stefan Leijnen:
Yeah. I agree with you there. I think the way you say it, it also relates to when we talked about business process and automation, right? So typically a person like that would come into a company to think about how automation or AI can help the company. But then you also need to have the personal skills to convince the rest of the company to change their business process. And that’s often very … It’s not just a technical skill, let’s put it that way. Camilla-
Camilla Olson:
So-
Stefan Leijnen:
Yeah.
So this, I’m really intrigued by this part of the conversation. Because I’m reflecting on my co-founder, Nick Clayton. Who I wished were here on the panel. And you’ll be amused by how this came about. He did children’s theatre with my son. So that’s how I met him. And so I think he was the smartest kid in Palo Alto. And went on to school, and whatever. And graduated in … Somehow recruited him to do this. And he’s just a genius. He’s great. But he’s the most ethical guy. But I don’t think of this as a data company at all. And so I need to talk to him about it, to see am I off somewhere else. Because I’m not a technologist. But you’ve all recognized that right away. But we think of it as technology, but not data.
But in terms of him leading it, I have to say we’re successful because he is … He’s incredibly smart and curious. But he’s also quite capable of putting himself in someone else’s shoes. And somehow he can do that with fashion designers. And I don’t know how he’s able to do that. But he’s able to do that.
Stefan Leijnen:
So Camilla, you mentioned a little bit earlier than I just stepped over the investment and the funding thing. Which is actually a good point. So maybe we should get back to that. And we have the following question. So to what extent would you agree that an investment in AI is paramount to the ongoing survival of your business? So in what ways can AI impact the competitive edge in the diversification of your products?
Camilla Olson:
For us, it’s a 100% of our business. It is-
Stefan Leijnen:
A 100%.
Camilla Olson:
… our business. It’s 100% of it. We wouldn’t have this company without it. It’s 100%. So is that … That’s the only way I can answer it. Would you like … I don’t know how else, what else I could say.
Stefan Leijnen:
Perhaps one of the other panelists would like to reflect on this, the investment in AI as a necessity for the ongoing survival of your business.
Sanjay.
Sanjay Mehra:
Yeah. I share this from let’s say from an apparel footwear consumer brand perspective, just as an example. I think it is ultra-critical to think about investment. I think equally important is to figure out how to do it in a smart way. So what I’m getting at is that this journey entails a lot of learning. Even if you’ve got the best data scientists, and you’ve got the best business experts, et cetera, et cetera. There is a ton of learning. And so I think the smart way to do this is to figure out how you can very rapidly get to some ROI, some outcomes that demonstrate the value. And can also act as a flywheel to get more momentum within the organization.
So thinking about the investment is not so much the amount. Because you can get a lot of benefit from a relatively reasonable amount, if you’re let’s say, for instance, partnering with a SaaS provider. I would throw our company in that space. But you can also go down and say, “I’m going to create my own data science capabilities. And make a massive investment in people and technology,” et cetera. So it’s about the ability to start small and to radically improve the speed that I think gets the best outcome.
Max Metral:
I have a comment to make. I obviously can not speak for the apparel industry, which I don’t know enough. But I have more of a general comment to make on how AI is to be shaping businesses nowadays. I think it’s not that it’s putting anyone at risk of [inaudible 00:48:34] business from one day to the other. It’s more to use the comparison of the arrival of electricity centuries ago. People were doing business before it was there. And it took some time for it to transition. But anyone who didn’t invest early enough [inaudible 00:48:50] enough. They were at risk of not being there in 20 or 30 years down the road. And it was not putting any imminent risk for the business to stop making business from one day to the other.
I think it’s the same with data. No one’s going to close shop tomorrow because of the lack of it, or at least not that I’m aware of. But if you don’t start at some point within the next few years, if you don’t get it right, you may be at risk of being overtaken by other companies that are smarter and better. And you might miss the point on many occasions.
Stefan Leijnen:
So let me put you on the spot with this question. Which is more important for a startup, funding or data?
Max Metral:
Funding to get the data right.
Stefan Leijnen:
I’m going to do a little round. I’m going to ask every one of you to answer the question, if you want to.
Sanjay Mehra:
I agree with Max.
Stefan Leijnen:
Okay.
Max Metral:
I mean, I’m obviously not an expert in this. I’m happy to follow Camilla and Sanjay’s feedback on this. But I believe that it’s about the idea where to do team you have together. And if you do things right, the funding should follow if you have a good idea, a good project, and the right people. So I’d maybe say even never easy, as Camilla said. But if you get the data or the mindset more than data, more the people around it, and the idea, the project, everything should follow hopefully.
Stefan Leijnen:
All right. So the better question will be funding data or talent, maybe then. I’d like to perhaps close off this panel. I’m looking if there are questions from the audience. I don’t see any. With a very open question that I’m hoping all of you would like to answer. Which is about the following, that it’s very difficult of course to predict the future. And it is particularly true for the future of predictive algorithms. But we can try. So what I’d like to reflect you on is, how do you see the future of AI in the apparel industry? And you can give a very general answer to the entire industry, or just for your company, which is also fine. But what I’m looking for is long term perspectives. And don’t worry, we won’t hold you to your promises in five or 10 years from now.
Max Metral:
I’m happy to start since I guess I’m the one who know the least on the subject.
Stefan Leijnen:
All right, Max.
Max Metral:
Based on what I’ve heard and based on the importance of the creative process, I’d say for me it’s how to combine the human and the AI into what the humans are good at, being creative. And AI is good at, being fast, computing the data in a very efficient and cost-effective way. I think the way to go is how you can combine the best of both worlds. What humans are good at, versus bad at. And how you can use computers to make them better at what they’re bad at. I think that’s how I would reply to the question.
Sanjay Mehra:
I just think back in history. And I look at the rise of computing, the rise of basic apps like Excel and Word. The rise of the iPhone, the rise of apps. I mean, these were all in their own ways breakthroughs. And we’ve come to live with them. And they are in many ways our best friends. And they have a lot of advantages, and they have some things that could be seen as a double-edged sword. And I think AI is going to be similar to that. It’s going to be our best friend. And we sometimes have to be watchful. So that’s my take.
Camilla Olson:
Do you want to go, Christiane?
Christiane Luible:
Yeah. But for me, I want to bring up the word for sustainability once more. And I think this for me now it’s the … I really hope that we find a good business model in the near future to get … It’s hard to act more sustainable. And the far future, I’m really curious. I’m really looking forward to the point when I really see, “Oh, wow. Yeah. Today, now it’s not just creating. It’s creativity.” So we really do something with the AI. And I don’t see it … I’m not frightened, but I think it’s interesting. But for the near future, definitely, sustainability, to find better solutions.
Camilla Olson:
So in the first quarter of next year, we’ll be able to go from the original design, original design details digitally from inspiration through to cut and sewn garment in less than an hour. And I mean, we’re partnering with people who can do. And applying that to on-demand manufacturing. And I think that that’s going to be just a game-changer in fashion. And with the ability to personalize trends for influencers, and individuals. I think the whole idea of personalized fashion is going to … Can easily become pervasive. And with on-demand manufacturing, this whole idea of waste is going to greatly be reduced. So I think AI is going to … And that’s only because AI is becoming cost-efficient, and making it possible.
Stefan Leijnen:
Thank you for those perspectives. I’m taking a quick peek at the audience’s questions. I have one more question. We have a couple of minutes left. So let me ask you this question, which is very relevant to the one that we’re in. Do you see the pandemic having any impact on AI implementation?
Sanjay Mehra:
Yes. We’ve seen customers focus of course on the e-commerce operations as everybody knows. And the openness to deploy and implement new AI based capabilities is extremely high. And what we’re seeing is, especially if you can focus on the near term, and share how you’re going to create a return on investment, let’s say within the three months timeframe. That is incredible. And so we are seeing a significant rise in demand.
Stefan Leijnen:
Is there anybody else who would like to reflect on this question? I don’t see hands raised.
Camilla Olson:
I think it has changed the industry. Because what we’re talking about doing, the on-demand manufacturing and all of that was two, three years out. And now we see that happening, we moved that forward two years in our planning. So it’s just brought everything forward in time. Like you’ve said, it moved e-commerce up, that growth significantly.
Stefan Leijnen:
Yeah. I think that’s actually very hopeful and optimistic perspectives that you just offered there at the final end. I think we’re also reaching the end of our panel discussion very shortly. I myself tried to weave in some of my own perspectives into the question asking. I didn’t offer too many answers myself. And that’s because I’m going to give a talk later today, which I’m now shamelessly promoting. For this panel, I’d really like to thank you, Christiane, Camilla, Sanjay, and Max. And of course the audience for joining us. I hope this has been very insightful. It was at least for me. So we have perhaps a two minute break. I don’t know the program exactly. And then I think the program will continue. So thank you very much for watching, and thank you for joining us all.
Sanjay Mehra:
Thank you, Stefan.
Christiane Luible:
Thank you.
Max Metral:
Thank you.
Stefan Leijnen:
Thank you.
Camilla Olson:
Thank you.