The Future of Competitive Price Matching in Retail
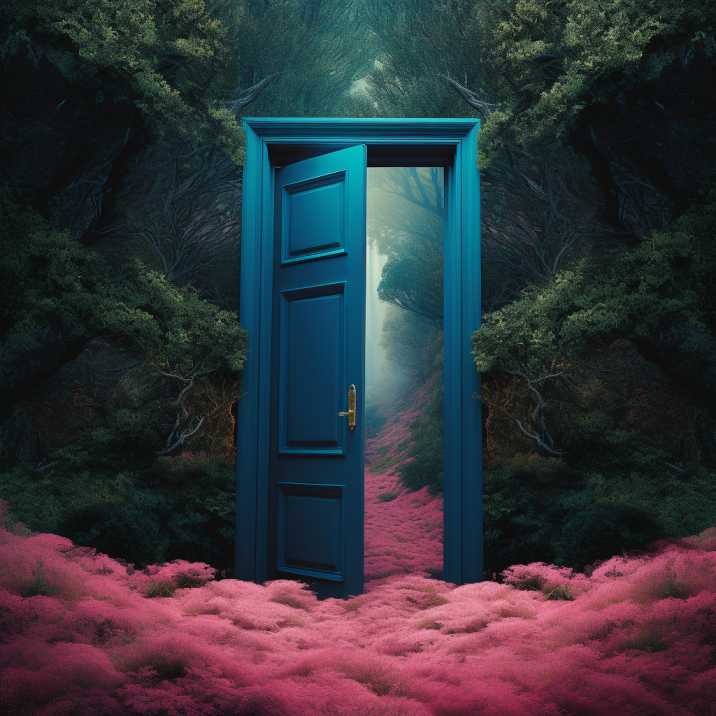
In the complex world of retail, product and price matching can be a daunting task, especially in categories lacking unique product identifiers. Various methods have been employed to tackle this issue, including the use of big data and AI techniques. However, these approaches often overlook a fundamental aspect: the consumer-demand angle. Traditional matching strategies focus on what’s available in the market, neglecting to consider the demand for a competitor’s product.
Why match a product’s price if the competitor’s demand is low? Conversely, high demand might necessitate a different course of action. The challenge lies in accessing this private demand data. If we could accurately match products while also understanding competitor demand, it would revolutionise decision-making in retail. This article explores this exciting new frontier in competitive price matching, offering insights into a future where pricing strategies are not just reactive, but also proactive and demand-driven.
Competitive Price Matching Methods
In the rapidly evolving retail landscape, competitive price matching has become an essential part of pricing strategy to stay relevant with consumers. Here are some key methods:
- Artificial Intelligence (AI) and Machine Learning (ML): AI and ML are transforming competitive price matching by analyzing vast amounts of data to predict consumer behavior. This enables more accurate and dynamic pricing strategies, such as predicting how a change in price will affect demand or identifying the optimal price for a product at a given time.
- Real-Time Pricing: Advancements in technology have made it possible to adjust prices in real-time based on current market conditions. Competitive price matching can now involve changing prices throughout the day based on factors like demand, competitor prices, and inventory levels.
- Personalized Pricing: With more customer data available, businesses can offer personalized prices as part of their competitive price matching strategy. This could involve offering discounts to price-sensitive customers or charging higher prices to those willing to pay more.
- Blockchain Technology: Blockchain could potentially revolutionize competitive price matching by creating a transparent and secure record of pricing data. This can prevent price manipulation and ensure fair competition.
- Predictive Analytics: Predictive analytics can forecast future price trends and consumer behavior, helping businesses plan their pricing strategies and stay ahead in competitive price matching.
- Integration of External Factors: Future competitive price matching strategies might consider a wider range of external factors, such as economic indicators, weather patterns, or social media trends, to adjust prices and stay competitive.
- Ethical Pricing: As consumers become more socially conscious, ethical considerations may become a part of competitive price matching strategies. This could involve ensuring fair prices for suppliers, considering the environmental impact of products, or offering discounts to low-income customers.
Competitive Pricing Strategy Construct
The competitive pricing strategy can be simplified into a straightforward framework for decision making. This framework consists of four quadrants, defined by two axes: competitive intensity and price sensitivity. However, a critical question arises: how can one gauge the competitive intensity of a product or similar products without understanding demand? Most existing solutions capture readily available competition data, providing insights into supply intensity but leaving demand intensity unaddressed. This is where Stylumia’s demand sensing engine comes into play. It not only captures the supply of competition but also gauges the demand of the market at the SKU level. This unique capability allows you to leverage the framework to its fullest potential, enabling strategic actions that are informed by both supply and demand dynamics.
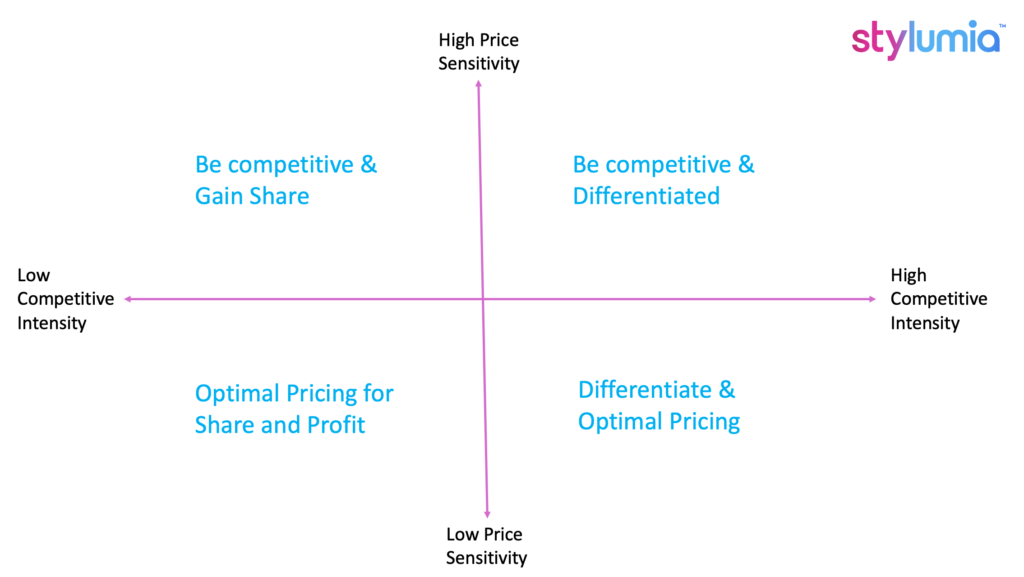
The quadrants of the framework are as follows:
- High Price Sensitivity, High Competitive Intensity: In this quadrant, customers are very sensitive to price changes and there is high competition. The focus should be on maintaining competitive prices and differentiating your product or service based on factors other than price, such as quality, service, or brand reputation.
- High Price Sensitivity, Low Competitive Intensity: Here, customers are very sensitive to price changes, but there is low competition. This could be an opportunity to capture market share through competitive pricing, but it’s also important to maintain profitability.
- Low Price Sensitivity, High Competitive Intensity: In this quadrant, customers are not very sensitive to price changes, but there is high competition. The focus should be on differentiating your product or service based on factors other than price. You may have more flexibility to set higher prices, especially if your product or service offers unique value.
- Low Price Sensitivity, Low Competitive Intensity: Here, customers are not very sensitive to price changes and there is low competition. This is an ideal situation where you have the opportunity to set prices that maximize profitability.
By understanding both the supply and demand dynamics of your market, you can navigate this framework more effectively and make strategic pricing decisions that drive success.
Understanding Pricing Sensitivity
Understanding price sensitivity, especially when product price changes are sparse, poses a significant challenge in competitive price matching. Various methods can address this issue, but incorporating competitive demand data can be particularly beneficial.
In scenarios where price alterations are infrequent and there’s a lack of substantial price change data, calculating price elasticity directly becomes a daunting task. However, several strategies can be employed to navigate this challenge:
Market Research and Surveys: Market research or surveys can be instrumental in understanding how customers might react to price changes. For instance, customers can be asked about their likelihood to continue purchasing a product if its price were to increase by a certain percentage. This approach can provide valuable insights for competitive price matching.
Conjoint Analysis: Conjoint analysis, a survey-based statistical technique used in market research, helps determine how people value different attributes of a product or service. It can be utilized to estimate potential changes in demand due to price changes, thereby informing competitive price matching strategies.
Experimental Price Changes: If feasible, implementing experimental price changes can be a viable strategy. This could involve altering the price of the product in a controlled setting and observing the change in demand. Such an experiment could be conducted in a specific store location or with a selected subset of customers.
Competitor Analysis: Examining similar products in the market and the impact of their price changes on demand can provide insights into how demand for your product might shift with price. This analysis is a crucial component of competitive price matching.
Econometric Models: Econometric models can be used to estimate price elasticity. These models can factor in a variety of elements, including price, income levels, tastes and preferences, and the prices of related goods. This complex approach typically requires the assistance of a trained economist or statistician. For more information on econometric models, you can refer to this link.
You can check how Stylumia’s demand-sensing engine captures the demand of the market/competition in the blog here. The technology is category agnostic while the blog largely talks about fashion.
In conclusion, competitive price matching is not just about matching or beating the prices of competitors. It’s about understanding the market, the consumer, and the various factors that influence pricing decisions. If you would like to get a sneak preview of what is possible using the future of competitive price and product matching, reach out us for a free consulting session here.