Unboxing Machine Learning Adoption In Fashion Retail
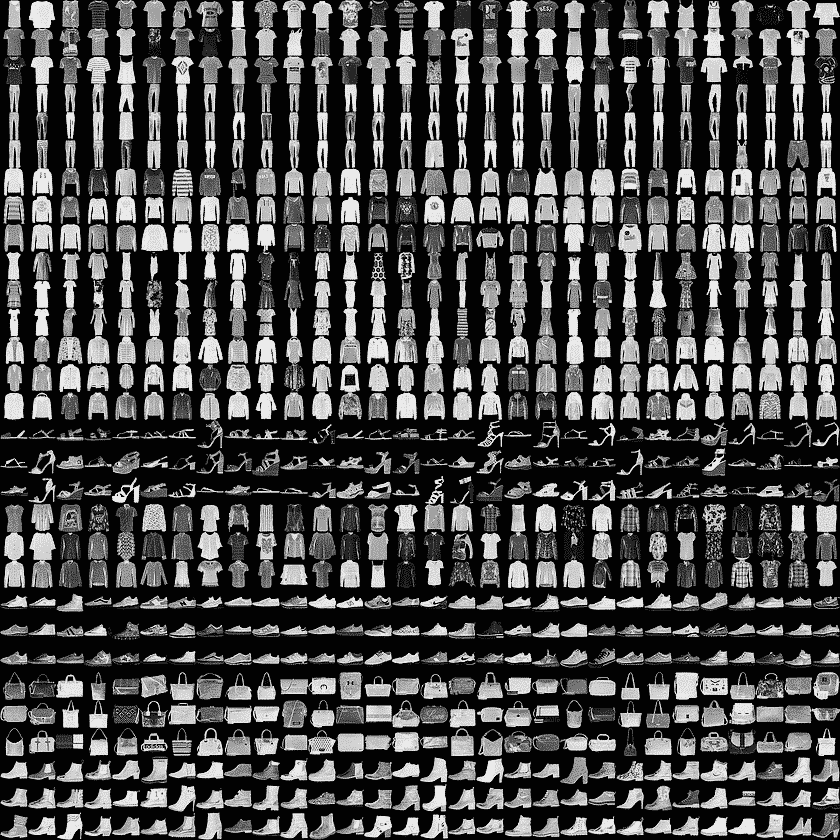
There is no business benefit without the adoption of technology. It’s also true for getting significant benefits through machine learning adoption in fashion retail. In this edit, we look at when is machine learning relevant or not and, what does it take to adopt for maximum benefit.
The purpose of machine learning in general is to detect patterns. The ability to detect patterns gives us the ability to predict better. There is so much prediction happening in fashion retail and hence understanding machine learning adoption in fashion retail is critical.
When Is Machine Learning Relevant?
Before we move into machine learning adoption in fashion retail, we need to understand when is ML relevant to solve your business problems.
Machine learning is relevant to solve a business problem when your problem,
- Has a complex logic
- Scales-up fast
- Requires specialized personalisation
- Adapts in near real-time
and when your data
- Can be used (available and accessible)
- Should be used (respects privacy & secure)
- Is high quality ( relevant, representative, fresh and unbiased)
When Is Machine Learning NOT Relevant?
Machine learning is not a magic wand for all problems. It is not relevant when your problem,
- Can be solved by simple rules
- Does not adapt to new data
- Requires 100% accuracy
- Requires full interpretability
Need Of Machine Learning Adoption In Fashion
We covered the need for a deep science to understand consumer demand in our earlier articles here and here. Considering when ML is needed as explained above, many fashion processes below meet the criteria through challenging problems.
- Fashion forecasting
- Trend validation
- Demand prediction of unseen products
- Demand planning for future seasons (OTB)
- Allocation planning & Execution
How Are Machine Learning Implementations Different
Data is the soil for any machine learning-based implementation. Unlike rule-based systems, machine learning systems need good preparation for data. It is like nurturing the soil to see a harvest at a later time.
Context of the data and interactions between the technology vendor/team and the brand users are very important. It will otherwise be garbage in garbage out. A rushed exercise will be futile without going through what it takes to do this stage effectively.
A false conclusion without careful implementation can be detrimental to the belief in technology and all the possibilities that the technology can provide for the medium to long term.
The economic benefit of getting planning accuracies is so high that it is worth spending a good time in this phase.
The following quote says it all for the essential ingredient of making ML projects successful.
Machine learning is all about data
Data is all about people
Machine learning is all about people
It is not just about the data, all the intuitions and domain intelligence in the business needs to be converted to data. The collective intelligence from data plus the converted domain intelligence makes the soil fertile.
It is a very collaborative and time-intensive effort to nurture the data. This includes cleaning and augmenting for anomalies and feature engineering.
The entire process is 80% data and 20% model. The models for fashion need to understand beyond textual attributes. That is incomplete data. This is non-trivial as the traditional models just take textual attributes which are insufficient to explain fashion. For eg. how do you articulate the exact color in language?
Another question is how do you choose the right machine learning strategy.
Choosing The Right Machine Learning Strategy
As expressed by Andrew NG, a thought leader in AI, the performance and data scale helps decide the right machine learning framework.
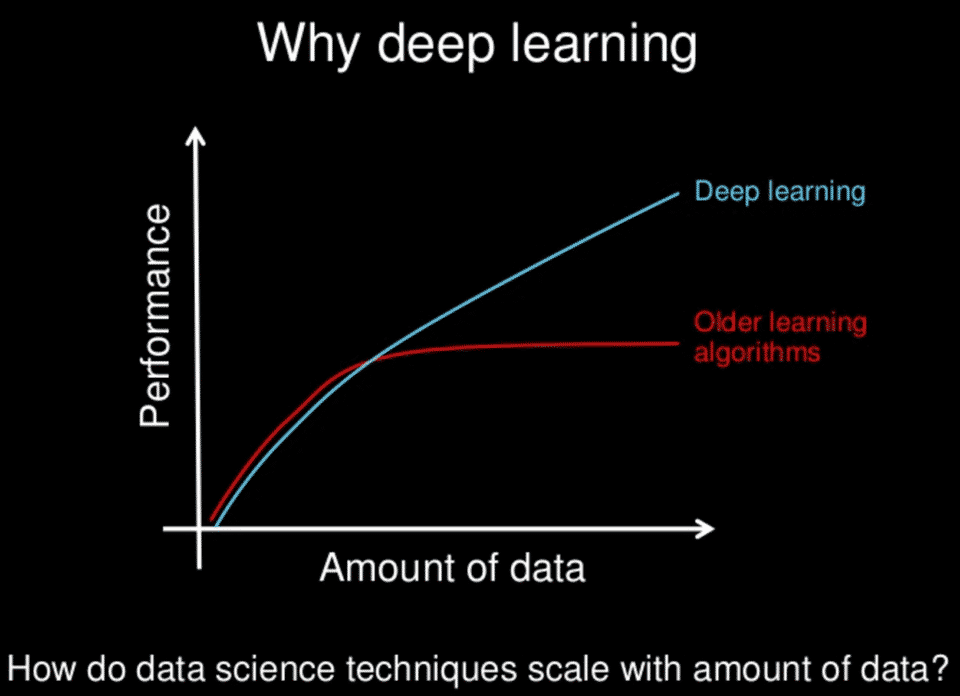
Build deep learning-based models when traditional models do not help you achieve desired accuracies and data scale also supports that. It will also help you assess the benefit you are getting over the traditional models.
For your brand to be fitted with a robust learning model, there is a need to sweat with data.
Sweat With Data
In our experience with clients, setting the right expectations at the beginning is critical to how the process will go. It has been ongoing learning for us too.
Shifting from rule-based systems to non-rule-based systems needs a shift in thinking.
We at Stylumia take extraordinary care in sweating with data. We at times rather take a few weeks more here than rushing. It has always been very productive. We are building a predictable consumer-demand engine for the brand. Also, the process goes through iteration for improvement in accuracy every time making the model align to brand DNA with the consumer.
We get an industry-leading accuracy lift from the baseline for brands across the value chain impacting close to doubling profitability.
Our clients appreciate this when they effectively see the lift and strong predictive muscle is built for the brand through sweating through the data work-out.
One of the questions that keep coming up globally is the interpretability of results. Here is a view on that.
How Much Does Interpretability Matter?
Interpretability is to know how the model has come out with certain decisions. While there are some ways to interpret (check this article for interpreting vision models), the challenge is in deep learning models. The construct you may use is to see if the benefits of the improvement far outweigh the need for interpretability, you should go ahead with the model that gives you significant lift. Scientists sometimes call this the black box fallacy.
In Conclusion,
Choose the right adoption framework and take extraordinary care in the data gathering, cleaning, enriching, and validation processes, for a successful machine learning project for your brand.
If you would like to make your brand a learning one, step jump in your forecast and planning accuracies through the value chain, you can reach out to us here.
Title Image Courtesy: Towards Data Science