How To Achieve Maximum Return On Fashion AI Projects
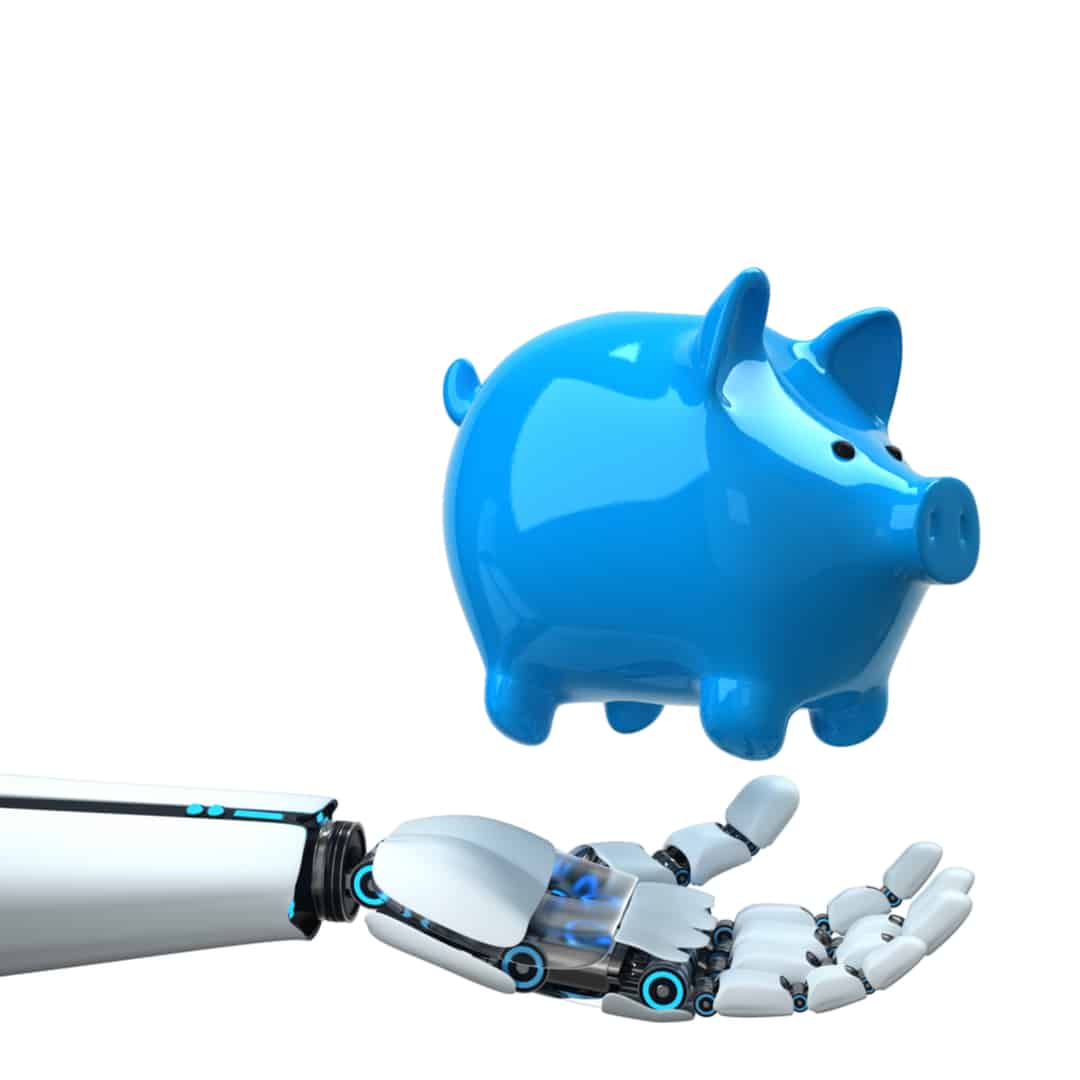
Return on fashion AI projects that you undertake is more critical now than ever. A recent study by Thomas H. Davenport (President’s Distinguished Professor of Information Technology and Management at Babson College, a visiting professor at Oxford’s Saïd Business School, and a fellow of the MIT Initiative on the Digital Economy) suggests a six-pronged approach to generate value. The value you are generating out of an AI project depends now on the “What” and the “How”. We see a good consistency of this framework and our experience in implementing AI projects with brands and retailers globally. Our earlier article on “Radical Analytics Adoption Framework” captures some essence of this.
Moreover, a good number of companies fail to realize the benefits from their AI initiatives due to the adoption framework. Detailed research by MIT/BCG throws some insights. The pioneers are the ones who smartly combine strategy organizational behavior and, technology. This edit provides a simple framework to give your projects a high probability of getting them right the first time.
Six Levers To Achieve Return On Fashion AI Projects
The role of executing AI projects may rest with different stakeholders across companies. In some companies, we have seen it owned by the analytics team, in some data science teams, in some innovation teams, etc. Irrespective of who owns it, the following framework helps you achieve a high probability of success.
# 1 Focus On Partnership With AI-friendly Business Units
Partnership with the business unit is imperative to any technology initiative’s success. In this case, choose a business unit that is technology or data or analytics friendly. One way is to look for leaders who are familiar with data, have curated data, and/or, have an analytics team with them.
In our implementation experience with brands and retailers, this is the first step that we take care of. We form a project team with this partnership in mind. It has almost always worked for us in taking the project to value addition.
In one of our implementations for an omnichannel brand, the VP of merchandising is an enthusiastic user and believer of technology who drives the initiative. In another multinational brand, the analytics head leads the project. We have cases where the head of buying and merchandising owns the transformation project.
# 2 Select Projects With Tangible Value
It is important to select projects which promise tangible value. Doing pilot alone is not enough but a clear path to implementation is critical. For example, segmenting customers alone is of no value. The value is on the application in personalization.
We follow a process of pilot – thin slice – full-scale approach to all our demand prediction implementations. A clear path to full-scale implementation exists from the word go. There are clear parameters at each stage gate for progress. The value brands get is the release of excess working capital, revenue lift, and profitability growth anywhere between 30-60%. In a recent implementation, we avoided a surplus investment of USD 5 million in under 6 months of engagement in a USD 150 M brand.
# 3 Get Stakeholder Trust And Partnership Early
As any data-led project involves effort, it is important to take the buy-in pre-engagement or development. One way to get this structured is to discuss the business use cases, prioritize them. Also, put down the cost and benefits of the options.
In a recent engagement, we discussed opportunities for pre-season demand forecasting, in-season management, and distribution optimization choices. The stakeholder prioritized in-season as the priority over the others considering lots of inventory in the system and that being a burning problem. We went ahead with in-season optimization as the first use case. We are now progressing to other use cases after a successful adoption of in-season.
# 4 Choose Reusable AI Projects
Considering the time and effort involved, choose projects which can be replicated across different categories and business units. This presents a scaled adoption and benefits opportunity after the first successful implementation.
This is very true in our implementations too. We choose a category in a brand as a starting point. In addition, after successful implementation, we adopt it across categories in a brand and then scale across brands. The approach provides smart resource deployment and ROI (return on investment) outcomes.
# 5 Use POC’s To Demonstrate Value
POC’s get you a roadmap to value generation but use them with a clear path to implementation. This will prevent full-scale deployment of high-cost/low-value projects.
We have seen in prediction projects, it is important to establish baseline shift inaccuracies compared to existing systems to build confidence and the value proposition. It is our default way of implementation and has helped establish value potential in full-scale implementation. Above all, it helps to minimize the effort of testing out new ideas.
# 6 Manage Pipeline To Full Implementation
While POC’s help with the start, as mentioned earlier, having a clear view of full scale is important. This includes the project pipeline from POC to full scale. Along with that, any communication, change management initiatives need to be planned. The focus to be always on adoption.
We believe in over-communication than less. Stakeholders are busy in retail. Having a scheduled rhythm with key stakeholders through the project roadmap has helped us in getting on-ground adoption-related feedback early.
In Conclusion,
AI application is promising to generate significant business benefits. In order to gain optimal value, it is important to have a structured approach to executing the project. The “How” of execution is as important as the “What” of the project. Those who succeed are the ones who get the What and the How right.
Hope this article gave you a research-backed perspective with case studies on how you can approach your advanced AI projects and gain significant business value.
If you are interested to know more about this, you can book a free consulting call with us here.